Recently, Reuters reported that OpenAI is considering developing its own chips. According to reports, since last year, OpenAI has begun to consider countermeasures for the shortage of artificial intelligence model training chips (that is, Nvidia GPU supply is tight), and is currently actively preparing to develop self-developed chips to meet future demand for artificial intelligence chips. . In fact, not long ago, OpenAI CEO Sam Altman publicly stated that Nvidia GPU shortages have a great impact on OpenAI and the entire artificial intelligence industry. In addition, starting this year, OpenAI began to recruit hardware-related talents. There are several software and hardware co-design positions recruiting on the official website. At the same time, in September this year, OpenAI also recruited Andrew Tulloch, a well-known expert in the field of artificial intelligence compilers. This seems to be It also confirms OpenAI’s investment in self-developed chips. OpenAI officially refused to comment on the matter, but if this matter finally comes to fruition, OpenAI will be the only Silicon Valley technology giant to join the ranks of self-developed chips after Google, Amazon, Microsoft, Tesla, etc.
Metrans is verified distributor by manufacture and have more than 30000 kinds of electronic components in stock, we guarantee that we are sale only New and Original!
As mentioned before, the main motivation for OpenAI's self-developed chips is the shortage of GPUs. More specifically, it is because whether it is purchasing Nvidia GPUs or using GPU-based cloud services, the price is too expensive, especially considering that the computing power required for OpenAI's future model training may increase exponentially.
OpenAI has been laying out generative artificial intelligence a few years ago. After the announcement of GPT-3 last year and ChatGPT in the second half of last year, the capabilities of these generative large language models have been greatly improved in the past few years and have reached the point where they can To the point where it can achieve meaningful dialogue with humans, OpenAI has become a leader in the field of artificial intelligence, and generative artificial intelligence has become the technology expected to have the greatest impact on human society in the next few years. According to Reuters, OpenAI recorded revenue of US$28 million last year and an overall loss of US$540 million. The main reason behind OpenAI’s huge losses is due to computing power expenses. It is worth noting that the loss of US$540 million was in 2022, the eve of the popularity of generative artificial intelligence; in the future, computing power expenditure is expected to increase exponentially, mainly due to:
- Competition for large models is more intense, models evolve faster, and the computing power required increases rapidly: In addition to OpenAI, technology giants such as Google are also pushing their own large models, which makes the evolution of large models significantly faster. It is expected that in the future A new generation will be updated every quarter to half a year, and the computing power required by the most cutting-edge models is estimated to increase by an order of magnitude every year.
- Large model application scenarios have become wider: Currently, Microsoft and Google have begun to use large models in the fields of search and code writing. It is expected that there will be more large model application scenarios in the future, including automatic task processing, multi-modal question answering, etc. , and these will greatly increase the number of different models, and also greatly increase the total computing power required for model deployment.
So, if OpenAI develops its own chips, how much cost will it save? Currently, the purchase cost of a server using an eight-card Nvidia H100 GPU is about US$300,000. Including the cloud service provider's premium, the total cost of using this server for three years is about US$1 million (this is the official quotation of AWS, others The prices provided by cloud service providers should be of the same order of magnitude); if OpenAI can use self-developed chips to reduce the cost of such an eight-card server to less than $100,000, its cost will be greatly reduced. On the other hand, if the self-developed chip is successful, it should be very promising to control the cost of a single accelerator card below US$10,000 in large-scale deployment. In other words, it is not possible to control the cost of an eight-card server below US$100,000. Out of reach.
What’s unique about OpenAI’s own AI chip?
At present, there are many technology companies that develop their own chips. So if OpenAI develops its own chips, how will it be different from the self-developed chips of Google, Amazon and other technology companies?
First of all, OpenAI's self-developed chips are purely for its own model training, which is different from the business models of Google, Amazon and other self-developed chips that are targeted at cloud servers for customers to use. For situations where Google, Amazon and other self-developed chips are used by cloud service customers, since the scenarios for users to use the model are not clear, the software stack used is uncertain, and the specific training model is also uncertain, so it is necessary to meet the requirements in chip design. Compatibility requirements, and such compatibility considerations often come at the expense of the efficiency and performance of each training task. On the contrary, OpenAI's self-developed chips are only for its own use, and the trained model is very clear: it is a large language model with Transformer as the basic component, and the software stack used is completely controlled by itself, so it can ensure that the design has a very high quality.
The second difference is that OpenAI has a very in-depth understanding of the model. OpenAI is a leader in the field of generative models. Currently, the GPT series models are still the best-performing models among large language generative models. Moreover, OpenAI has many years of accumulation in the field of generative models. Therefore, OpenAI has various design solutions for current generative models. Having an in-depth understanding means that OpenAI has enough capabilities and accumulation to do chip-model collaborative design, and can design corresponding models according to the characteristics of the chip. At the same time, it can also clarify the design indicators of the chip according to the needs of the model, including how to Make optimal compromises among computing units, storage and chip-to-chip interconnects, etc. The most important thing is that OpenAI has the clearest plan in the industry for the roadmap of generative large models in the next few years. This means that even if it takes several years to develop a self-developed chip, there is no need to worry too much about the fact that the chip will no longer be able to be mass-produced. Keep up with model updates. From this perspective, OpenAI’s self-developed chips are very different from Google and Amazon, but they are similar to Tesla’s Dojo series self-developed model training chips; but they are different from Tesla. What's more, OpenAI's demand for model training will obviously be much higher than Tesla's, and the importance of such self-developed chips will also be higher for OpenAI.
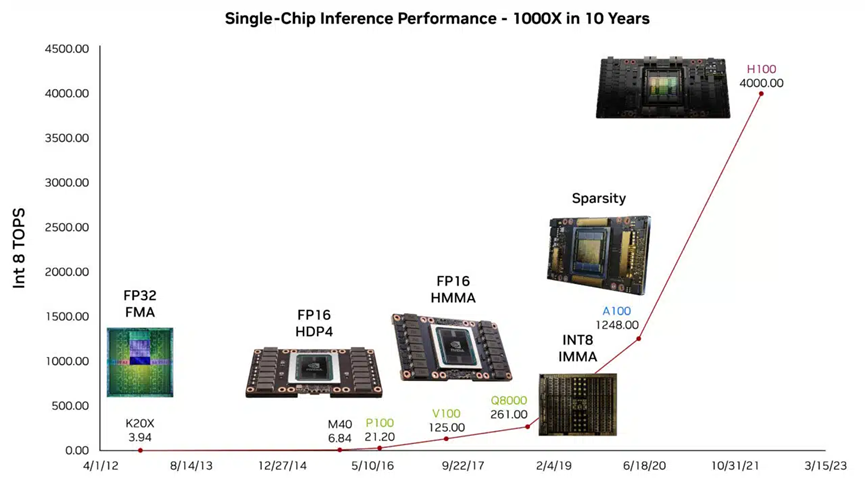
These unique features of OpenAI give it the opportunity to implement high-performance chips using unconventional specialized designs. Recently, Nvidia analyzed the performance improvement rules of its GPU in its official blog: Nvidia's GPU computing power has increased by 1,000 times in less than ten years. According to the analysis, in the 1,000-fold increase in computing power, the optimization of calculation accuracy (that is, using 16-bit or even 8-bit floating point numbers to replace the original 32-bit floating point number calculation) and use a dedicated computing module to achieve a 16-fold performance improvement, while the collaborative optimization of the chip architecture field and the compiler provides a 12.5-fold performance improvement. On the other hand, the performance improvement brought by semiconductor technology is only twice. It can be seen that in the field of high-performance computing chips, the co-design of algorithms and chip architecture (including model algorithms and compiler algorithms) is the main driving force for performance improvement (also known as Huang's Law). From this perspective, OpenAI is indeed in a very advantageous position. With its deep understanding of algorithms, OpenAI is expected to make full use of Huang's Law to realize the design of high-performance computing chips in the next few years.
Challenges of OpenAI’s own AI chips
In addition to its own advantages, OpenAI's self-developed chips also have challenges.
The goal of OpenAI's self-developed chips is clear, which is to use high computing power chips for large models. The primary challenge of high-computing chips is their complexity. From a chip design perspective, the computing units, storage access, and interconnections between chips in high-performance computing chips all need to be carefully considered. For example, in order to meet the needs of large models, chips will most likely use HBM memory; in order to achieve high energy efficiency and scale of chips, it is expected that advanced processes will be used with chip pellets and other technologies to achieve high yields; large models will usually use distribution For formula computing, the interconnection between chips is crucial (Nvidia’s NVLINK and InfiniBand technologies are very important for GPUs, and OpenAI also requires similar technologies). Each of these chip design components requires a team with considerable experience to implement, and integrating these components together also requires excellent architectural design to ensure overall performance. How OpenAI can assemble an experienced team to do these challenging designs in a short period of time will be an important challenge.
In addition to chip design, how to ensure that software and hardware work together, or in other words, how to design a high-performance compiler and related software ecosystem is another major challenge for OpenAI. Currently, an important advantage of Nvidia GPU is that its CUDA software system has high performance and compatibility after more than ten years of accumulation. In OpenAI's self-developed chip, the compiler system also needs to achieve high performance such as CUDA to fully utilize the computing power of the chip. Unlike other technology companies developing self-developed chips for cloud services, OpenAI's chips are mainly for their own use, so there is no need to worry too much about the ecology and user model support. However, the compilation performance also needs to be close to Nvidia's CUDA. OK. In fact, OpenAI has already invested in this field from earlier; in July this year, OpenAI announced its own artificial intelligence model compilation solution based on the open source Triton language, which can compile Python code into intermediate code using the open source Triton language. representation, IR), and then use the Triton compiler and LLVM compiler to compile to PTX code, so that it can run directly on PTX-enabled GPUs and artificial intelligence accelerators. From this perspective, OpenAI’s investment in compilers may be a precursor to its self-developed chips.
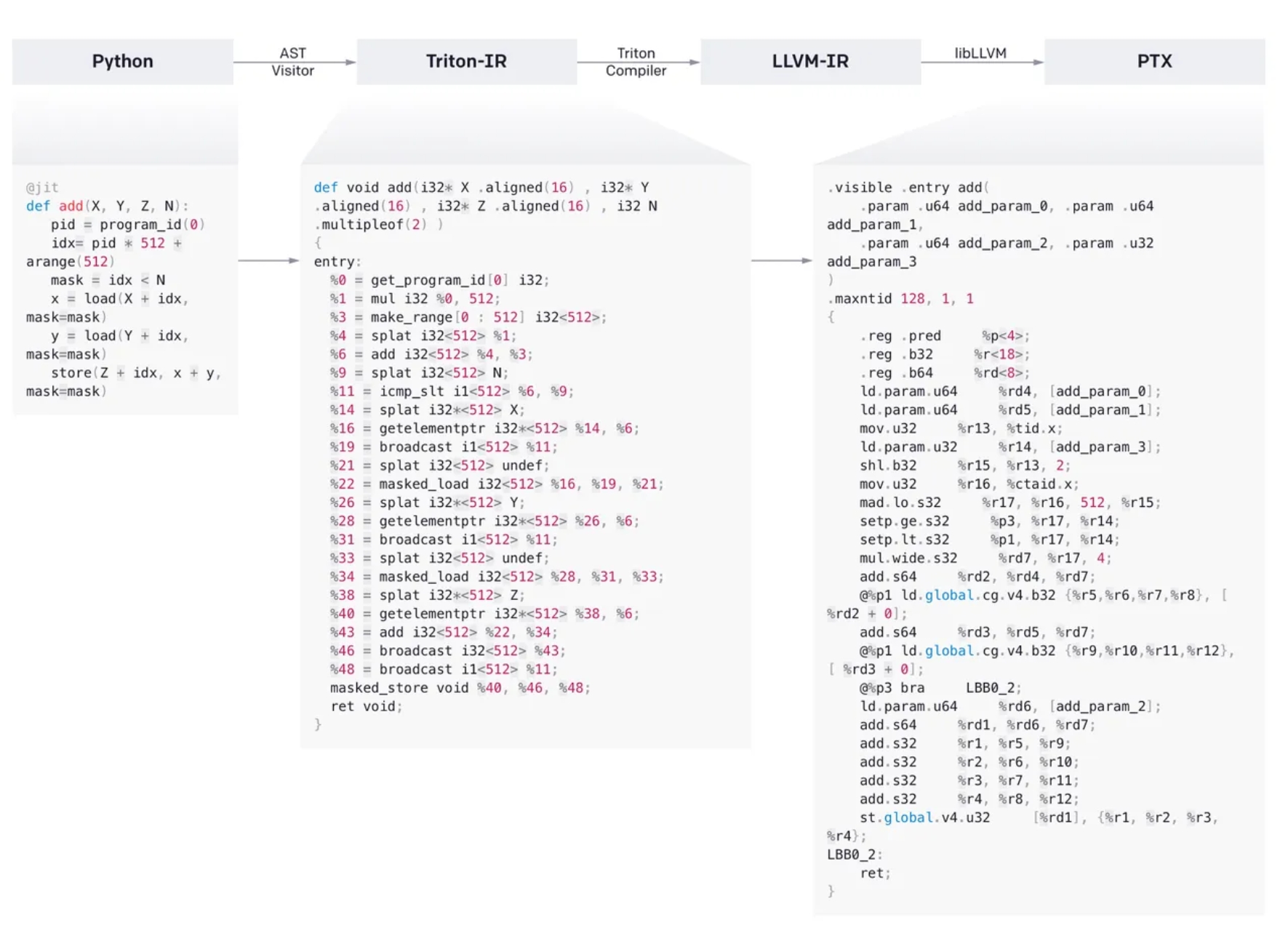
Finally, the specific production of chips will also be a challenge. As mentioned before, OpenAI will most likely be implemented using advanced process nodes and advanced packaging technologies. Therefore, how to ensure the yield of production, and more importantly, how to maintain production capacity in advanced packaging and advanced process nodes in a few years when the capacity may still be tight. Obtaining enough production capacity for mass production is also a problem that needs to be solved.
Considering these three challenges, we believe that OpenAI’s current plan to develop its own chips may be multi-step. First of all, before the technical team and production problems are completely solved, OpenAI can choose to cooperate with Microsoft (its largest shareholder, which also has its own chip plan Athena) and Nvidia (or AMD) to choose semi-customized chips. For example, OpenAI provides chip needs It supports some indicators and even provides some IP, and can work with these partners to design and produce chips. After the technical team and production problems are solved, OpenAI can choose to invest heavily in self-developed fully customized chips to achieve the best performance and controllability.